Improving the performance modelling of solar photovoltaics
Bin Meng defended his PhD thesis at the Department of Built Environment on June 25th.
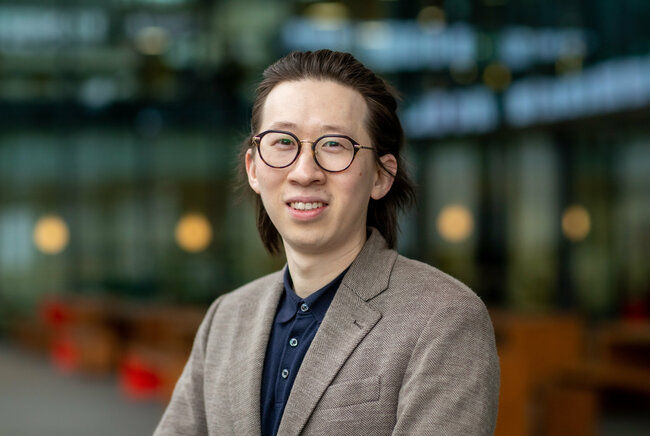
Distributed photovoltaic (PV) systems have received increasing attention in recent years, and accounts for nearly half of the newly installed capacity among the annual additional PV installations. PV performance modeling plays a critical role throughout the design phase, commissioning phase, and operation and maintenance phases of a PV project. However, there are several challenges that must be overcome to improve the modelling. For his PhD thesis, Bin Meng looked at ways to improve improve state-of-the-art performance modelling of distributed PV installations within the built environment.
Several challenges exist that must be overcome to improve the performance modelling of distributed PV systems in the built environment. First, there is a general lack of weather condition measurement in the field. Second, the complexity of actual operation conditions must also be considered, especially arbitrary shading conditions in built environment. Third, the simulation process can be hindered by the frequent occurrence of missing or misrepresented PV orientation information.
Improve state-of-the-art
For his PhD research, Bin Meng sought to improve state-of-the-art performance modelling of distributed PV installations within the built environment, with a focus on developing scalable fit-for-purpose methods tailored to various phases of the installations’ lifecycle.
To fulfill this research aim, Meng addressed several objectives. First, he developed a novel method to infer tilt and azimuth angles of distributed PV systems, utilizing widely available data. Second, he identified the performance variability amongst identical rooftop PV systems in the field and formulated risk mitigation strategies to reduce the error of long-term yield prediction.
Third, he bridged the knowledge gap in the field of fault detection for distributed PV systems by constructing dynamic PV power benchmarks and cumulative sum charts to monitor PV performance continuously based on machine learning algorithms and widely available data.
Finally, he improved the probabilistic yield forecasting for distributed PV systems under local shading conditions by developing a hybrid method which combines physical model with data-driven model.
Widely applicable
The developed fit-for-purpose methods are widely applicable to the distributed PV installations, which eliminate the need for on-site weather measurements, relying instead on open-source weather data.
By holistically addressing the multifaceted existing challenges, Meng’s research encapsulates a strategy that paves the way for more efficient, reliable, and sustainable utilization of solar energy in the built environment.
Title of PhD thesis: Towards improved performance modelling of distributed PV systems in the built environment. Supervisors: Jan Hensen and Roel Loonen.