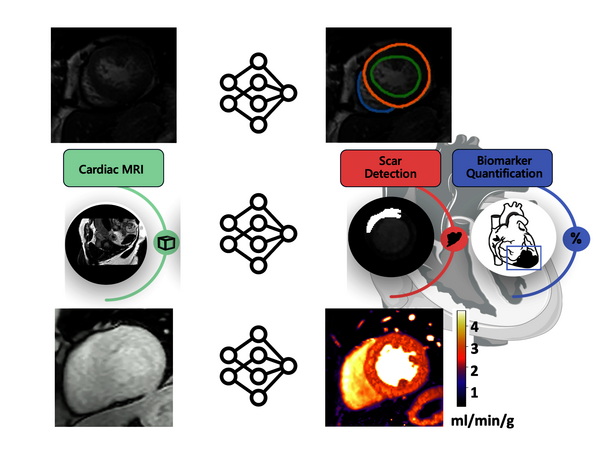
This research line aims to provide clinicians with a comprehensive assessment of the heart’s health in a quantitative and automated manner, using artificial intelligence (AI).
Cardiac magnetic resonance (CMR) imaging can provide insight into the anatomy, function and viability of the heart muscle (myocardium), and blood flow in the heart chambers (atria and ventricles) and surrounding large vessels. Each of these aspects is studied with a dedicated scan protocol, resulting in a large number of different images per examination. In clinical practice, these images are assessed visually which is a challenging and time-consuming process, requiring a high level of training.
We are working to automatically extract quantitative biomarkers of perfusion, scar, and the contractile function of the left ventricle from CMR data, using AI-based image analysis. We aim to combine the analyses to leverage the complementary information across the data. And we aim to combine the interpretation of these biomarkers to learn more detailed phenotypes of the myocardium and thus, give more personalised decisions, while also being fast, user-independent, and more accessible. We are also working to build the evidence required to support the clinical integration of these technologies and enable the transition of CMR imaging from expert use, only in primarily academic centers, to broader use in regional hospitals.
This research is performed in close cooperation with King’s College London, St. Thomas Hospital.
To achieve our aims, we are also working to make the following fundamental improvements to AI methods:
- exploring data-efficient (self-supervised, unsupervised,…) learning to exploit large unlabelled clinical databases.
- embedding domain knowledge and incorporating prior anatomical, physical, and clinical information in model architectures to improve efficacy.
- understanding when AI will fail, using out-of-distribution detection.
- leveraging generative AI with a view to using synthetic images in model training and improving the quality of clinical data.